Manufacturing and Heavy Industry operations around the world rely on their machinery to get the job done, efficiently and effectively. The cost of equipment failure and the resulting unplanned downtime has serious consequences for the bottom line, with medium unplanned downtime costs approximately $125,000 per hour. When inflationary pressures, supply chain demands and raw material costs are factored in, unplanned downtime costs for Heavy Industry were calculated as $59 million per year in 2023.
Faced with the need to minimize the business impact of unplanned downtime for critical equipment, industries with heavy assets and significant downtime costs, such as oil & gas and mining, are leading the way in adopting Predictive Maintenance solutions.
By incorporating satellite connected IoT sensors, Heavy Industries operating in remote locations can reliably monitor machinery in real time and react quickly to avoid equipment failures and keep assets operational. The data from satellite-connected sensors on equipment forms a vital component of deploying Predictive Maintenance programs in industries with high asset costs.
What is Predictive Maintenance?
Predictive Maintenance (PdM) is a proactive, data-driven approach that uses advanced technologies – such as condition monitoring, machine learning (ML) and IoT devices – to anticipate equipment failures and schedule maintenance before disruptions occur. By analyzing real-time data from sensors installed on machinery, PdM identifies early signs of wear, faults, or deterioration, enabling timely intervention to prevent costly downtime.
Unlike time-based or reactive maintenance, PdM optimizes equipment performance by triggering maintenance tasks only when specific conditions indicate a need. This approach improves equipment reliability, reduces maintenance expenses, and extends the lifespan of assets. AI-powered analytics and IoT-enabled sensors track key metrics like temperature, pressure or vibration, providing continuous insights into machine performance. When thresholds are exceeded, PdM systems issue alerts or initiate maintenance work orders.
The goal of PdM is to enhance operational efficiency by minimizing unplanned downtime, lowering maintenance costs, and ensuring asset reliability. Industries such as manufacturing, energy, and transportation rely on PdM to align maintenance activities with actual equipment conditions, maximizing productivity and supporting cost-effective, sustainable operations.
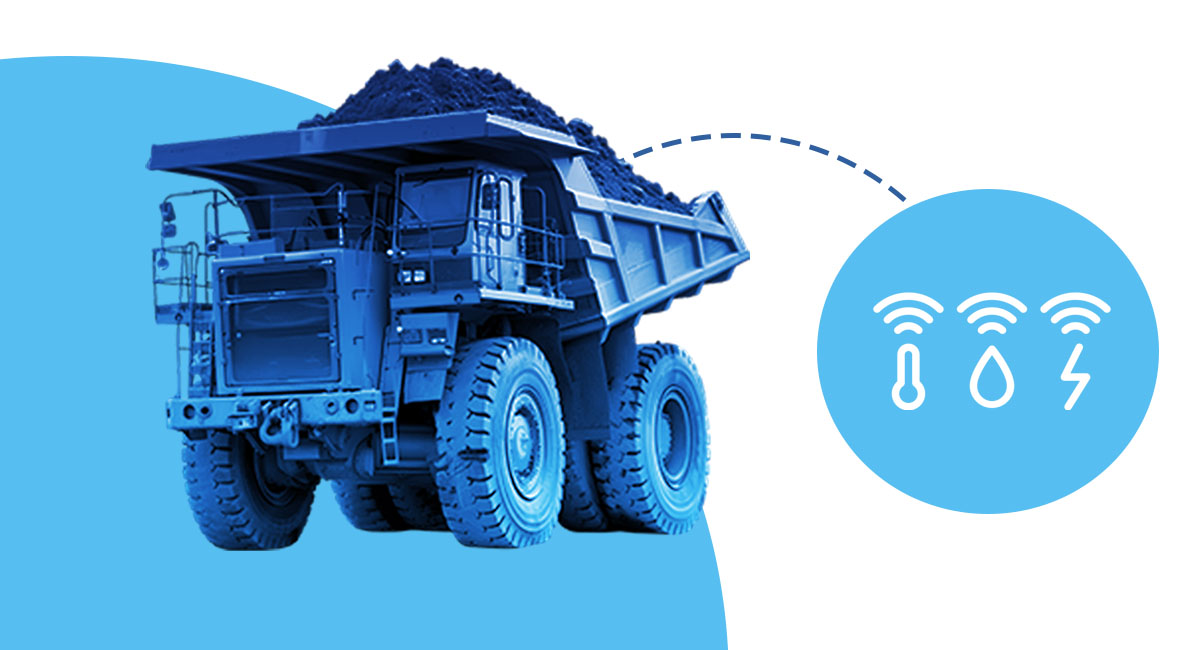
What is the Difference between Predictive and Preventive Maintenance?
Although often used interchangeably, Predictive Maintenance (PdM) and Preventive Maintenance (PM) are distinct approaches to equipment upkeep, each suited to different operational needs.
Preventive Maintenance follows a scheduled approach, performing maintenance at regular intervals based on time or measurable usage units, such as engine hours or production cycles. This method ensures equipment is inspected and maintained before issues arise, but it does not consider the actual condition of the asset.
For instance, a Mining operation may replace drill components every six months, regardless of whether those components show signs of wear. While this minimizes the chance of failure, it may result in premature replacements or unnecessary downtime.
Predictive Maintenance leverages real-time data from IoT sensors and advanced analytics to monitor the actual condition of assets. Maintenance is performed only when necessary, based on insights into potential failures or performance degradation.
For example, IoT sensors on a Combine Harvester may detect rising temperatures or irregular vibrations, indicating wear and tear. Predictive maintenance enables technicians to address the issue before a failure occurs, minimizing downtime and repair costs.
Comparing the Two Approaches
Types of Predictive Maintenance
There are three distinct types of Predictive Maintenance: Indirect Failure Prediction, Anomaly Detection, and Remaining Useful Life (RUL). Each approach differs in its desired objectives, the analytical methods used, and the type of information output provided.
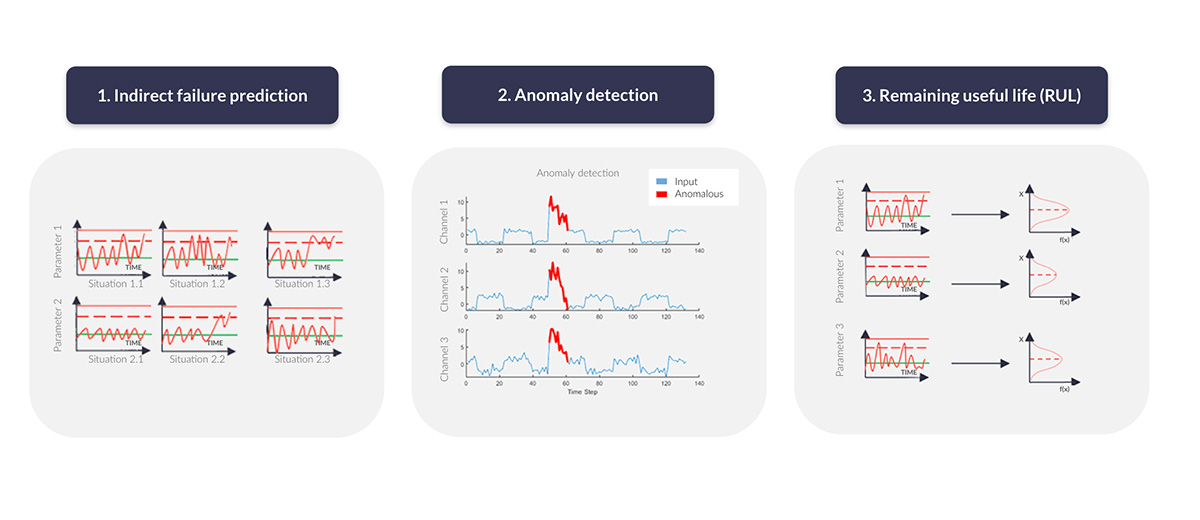
Image adapted from the IoT Analytics Asset Performance & Predictive Maintenance Market Report 2023–2028
Indirect Failure Prediction
Estimates equipment health by calculating a ‘health score’ based on known maintenance requirements, operating conditions and historical performance data. When sufficient data is available, supervised machine learning can be applied to refine the predictions. This approach is scalable since it relies on manufacturer specifications, and it is cost-effective because it uses existing sensors.
Its dependence on large volumes of historical data may render it unsuitable for industries like heavy machinery, where high downtime costs necessitate more immediate and accurate insights.
Anomaly Detection
Identifies potential failures by detecting deviations from normal operating conditions in real time. Unlike methods that require historical data, it relies on current sensor data, making it particularly suited to organizations without extensive machinery usage records. This approach improves predictive accuracy by considering real-time environmental and operational factors rather than predefined maintenance parameters set by the manufacturers.
The risk of false positives can pose challenges, as unnecessary alerts may disrupt operations and complicate machine learning algorithm performance.
Remaining Useful Life (RUL)
Focuses on predicting the time left before equipment failure based on specific machine metrics such as operational hours, distance traveled, or activity cycles. By analyzing sensor data, this method identifies condition indicators that highlight whether the equipment is performing as expected or if faults have accelerated its degradation. RUL models are trained using system data collected under known conditions and applied to predict outcomes under new or variable circumstances.
While this method is highly robust and reliable, it requires detailed, high-quality data for accurate predictions, making it particularly effective for critical equipment in complex environments.
The Benefits of Predictive Maintenance
Predictive Maintenance brings many benefits to organizations through its advanced approach to equipment upkeep, using technology and data analysis to improve asset reliability and efficiency. By identifying potential issues before they lead to failures, PdM helps organizations reduce downtime, optimize resources, and maintain safer working environments.
Research, including findings from the US Department of Energy, highlights the tangible impact of Predictive Maintenance. Compared to preventive maintenance programs, it offers cost savings of 8% to 12%, and when compared to reactive maintenance, cost savings increase to 30% to 40%. These programs also enable a reduction in maintenance costs by 25% to 30% and minimize equipment breakdowns by 70% to 75%.
In addition to cost savings, PdM improves operational efficiency by reducing downtime by 35% to 45% and increasing production capacity by 20% to 25%.
40%
Cost Savings
30-45%
Downtime Reduction
75%
Fewer Equipment Breakdowns
How to Implement Predictive Maintenance
1. Establish Baselines and Data Collection
Baseline performance metrics are identified for the assets by monitoring its condition to set the normal performance benchmarks. Once the baseline is established, sensors are installed to capture real-time data, enabling continuous performance monitoring.
2. Install IoT Sensors on Equipment
IoT sensors are installed on critical equipment to monitor various parameters such as vibration, temperature, pressure, and noise. These sensors continuously collect data on the equipment’s condition and the data gathered is then transmitted to a centralized system for analysis.
3. Data Integration and System Setup
The data collected from the IoT sensors needs to be integrated with the PdM system. This involves connecting the sensors to a computerized maintenance management system (CMMS) or a remote dashboard which allows for real-time monitoring and data analysis.
4. Set Maintenance Thresholds and Automate Alerts
Organizations need to define thresholds for acceptable performance levels. When these thresholds are exceeded, the system automatically triggers maintenance alerts, enabling timely interventions before equipment failure occurs.
5. Select and Implement the Right Analytics Tools
An analytics platform is required to handle the large volumes of data, apply predictive models, and generate actionable insights. Machine learning and AI algorithms are crucial for analyzing sensor data and predicting future equipment failures based on historical data.
6. Develop Predictive Models and Train the System
Predictive models are developed using historical data, maintenance logs and sensor data to forecast future equipment behavior. These models are trained to identify patterns in the data that may signal the onset of failure.
7. Integration with Existing Maintenance Systems
The PdM system is integrated with existing workflows, maintenance management systems, and enterprise resource planning (ERP) systems. This enables seamless communication across platforms and allows for data-driven decision-making.
8. Monitor and Optimize the Program
After implementation, the PdM program should be monitored to evaluate its effectiveness. Continuous data collection and model refinement will help improve prediction accuracy over time.
Industrial Applications of Predictive Maintenance
Predictive Maintenance is becoming increasingly common practice in asset-intensive industries that depend on their large, complex machinery. For industries with assets in remote locations or critical communication requirements, satellite connected IoT devices can transmit real-time sensor data for PdM programs.
Predictive Maintenance and Satellite IoT
For remote operations, such as those found in mining or offshore environments, Satellite IoT becomes a crucial part of the Predictive Maintenance Program. When assets are located in areas with unreliable or no cellular connectivity, traditional IoT solutions relying on cellular networks may fail to transmit vital data. Satellite IoT solutions overcome this challenge by enabling real-time data transmission via satellite, ensuring that assets can be monitored regardless of their location or environment.
Beyond just sensor data collection, Satellite IoT can enable remote control of assets. If an asset is detected to be operating in an unsafe condition, it can be remotely shut down to prevent catastrophic damage or safety incidents. This combination of real-time monitoring and remote intervention significantly enhances worker safety and helps avert equipment breakdowns before they escalate into more serious issues.
Get in Touch
At Ground Control, we design and build Satellite IoT devices leveraging the Iridium global network, providing reliable real-time data transfer from anywhere on Earth. Our feature-rich IoT platform, Cloudloop, can monitor and analyse sensor data and offers a simplified and well-documented API to connect to your existing Predictive Maintenance and Asset Performance Management (APM) toolkits.
With over 20 years of experience, we can help you make the best choices based on your requirements.